Research Article | OPEN ACCESS
Abnormality Segmentation and Classification of Brain MR Images using Combined Edge, Texture Region Features and Radial basics Function
1B. Balakumar and 2P. Raviraj
1Centre for Information Technology and Engineering, M.S. University, Tirunelveli, Tamilnadu, India
2Department of CSE, Kalaignar Karunanidhi Institute of Technology, Coimbatore, Tamilnadu, India
Research Journal of Applied Sciences, Engineering and Technology 2013 21:4040-4045
Received: January 26, 2013 | Accepted: February 25, 2013 | Published: November 20, 2013
Abstract
Magnetic Resonance Images (MRI) are widely used in the diagnosis of Brain tumor. In this study we have developed a new approach for automatic classification of the normal and abnormal non-enhanced MRI images. The proposed method consists of four stages namely Preprocessing, feature extraction, feature reduction and classification. In the first stage anisotropic filter is applied for noise reduction and to make the image suitable for extracting the features. In the second stage, Region growing base segmentation is used for partitioning the image into meaningful regions. In the third stage, combined edge and Texture based features are extracted using Histogram and Gray Level Co-occurrence Matrix (GLCM) from the segmented image. In the next stage PCA is used to reduce the dimensionality of the Feature space which results in a more efficient and accurate classification. Finally, in the classification stage, a supervised Radial Basics Function (RBF) classifier is used to classify the experimental images into normal and abnormal. The obtained experimental are evaluated using the metrics sensitivity, specificity and accuracy. For comparison, the performance of the proposed technique has significantly improved the tumor detection accuracy with other neural network based classifier SVM, FFNN and FSVM.
Keywords:
Classification, feature extraction, MRI, RBF, segmentation, tumor,
Competing interests
The authors have no competing interests.
Open Access Policy
This article is distributed under the terms of the Creative Commons Attribution 4.0 International License (http://creativecommons.org/licenses/by/4.0/), which permits unrestricted use, distribution, and reproduction in any medium, provided you give appropriate credit to the original author(s) and the source, provide a link to the Creative Commons license, and indicate if changes were made.
Copyright
The authors have no competing interests.
|
|
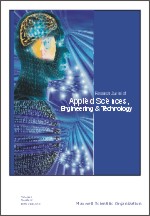 |
ISSN (Online): 2040-7467
ISSN (Print): 2040-7459 |
 |
Information |
|
|
|
Sales & Services |
|
|
|