Research Article | OPEN ACCESS
Neural Network Based STLF Model to Study the Seasonal Impact of Weather and Exogenous Variables
Muhammad Qamar Raza, Zuhairi Baharudin, Badar-Ul-Islam, Mohd. Azman Zakariya and Mohd Haris Md Khir
Department of Electrical and Electronics Engineering, Universiti Teknologi PETRONAS 31750 Tronoh, Perak, Malaysia
Research Journal of Applied Sciences, Engineering and Technology 2013 20:3729-3735
Received: December 26, 2012 | Accepted: March 14, 2013 | Published: November 10, 2013
Abstract
Load forecasting is very essential for efficient and reliable operation of the power system. Uncertainties of weather behavior significantly affect the prediction accuracy, which increases the operational cost. In this study, neural network (NN) based 168 hours ahead short term load forecast (STLF) model is proposed to study seasonal impact of calendar year. The affect of the model inputs such as, weather variables, calendar events and type of a day on load demand is considered to enhance the forecast accuracy. The weight update equations of gradient descent algorithm are derived and Mean Absolute Percentage Error (MAPE) is used as performance index. The performance of NN is measured in terms of confidence interval, which is based on training, testing, validation and cumulative impact of these phases. The simulations result shows that the forecast accuracy is affected by seasonal variation of input data.
Keywords:
Artificial Neural Network (ANN), calendar events, gradient based algorithm, Mean Absolute Percentage Error (MAPE), weather variables,
Competing interests
The authors have no competing interests.
Open Access Policy
This article is distributed under the terms of the Creative Commons Attribution 4.0 International License (http://creativecommons.org/licenses/by/4.0/), which permits unrestricted use, distribution, and reproduction in any medium, provided you give appropriate credit to the original author(s) and the source, provide a link to the Creative Commons license, and indicate if changes were made.
Copyright
The authors have no competing interests.
|
|
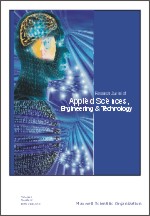 |
ISSN (Online): 2040-7467
ISSN (Print): 2040-7459 |
 |
Information |
|
|
|
Sales & Services |
|
|
|