Research Article | OPEN ACCESS
Handling Intrusion Detection System using Snort Based Statistical Algorithm and Semi-supervised Approach
G.V. Nadiammai and M. Hemalatha
Department of Computer Science, Karpagam University, Coimbatore-641021, Tamil Nadu, India
Research Journal of Applied Sciences, Engineering and Technology 2013 16:2914-2922
Received: December 10, 2012 | Accepted: January 11, 2013 | Published: September 10, 2013
Abstract
Intrusion detection system aims at analyzing the severity of network in terms of attack or normal one. Due to the advancement in computer field, there are numerous number of threat exploits attack over huge network. Attack rate increases gradually as detection rate increase. The main goal of using data mining within intrusion detection is to reduce the false alarm rate and to improve the detection rate too. Machine learning algorithms accomplishes to solve the detection problem. In this study, first we analyzed the statistical based anomaly methods such as ALAD, LEARAD and PHAD. Then a new approach is proposed for hybrid intrusion detection. Secondly, the advantage of both supervised and unsupervised has been used to develop a semi-supervised method. Our experimental method is done with the help of KDD Cup 99 dataset. The proposed hybrid IDS detects 149 attacks (nearly 83%) out of 180 attacks by training in one week attack free data. Finally, the proposed semi-supervised approach shows 98.88% accuracy and false alarm rate of 0.5533% after training on 2500 data instances.
Keywords:
Application Layer Anomaly Detector (ALAD), intrusion detection, KDD cup 99 datasets, Learning Rules for Anomaly Detection (LERAD), Packet Header Anomaly Detection (PHAD), semi-supervised approach, snort,
Competing interests
The authors have no competing interests.
Open Access Policy
This article is distributed under the terms of the Creative Commons Attribution 4.0 International License (http://creativecommons.org/licenses/by/4.0/), which permits unrestricted use, distribution, and reproduction in any medium, provided you give appropriate credit to the original author(s) and the source, provide a link to the Creative Commons license, and indicate if changes were made.
Copyright
The authors have no competing interests.
|
|
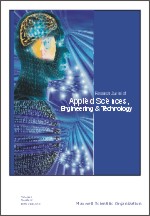 |
ISSN (Online): 2040-7467
ISSN (Print): 2040-7459 |
 |
Information |
|
|
|
Sales & Services |
|
|
|