Research Article | OPEN ACCESS
Econometric and RBF Neural Network Models for Analyzing Automobile Demand in Iran
1Hamed Pouralikhani, 1Alimohammad Kimiagari, 1Mohsen Keyvanloo and 2Hesamaddin Najmi
1Department of Industrial Engineering, Amirkabir University of Technology, Tehran, Iran
2Department of Industrial Engineering, Iran University of Science and Technology, Tehran, Iran
Research Journal of Applied Sciences, Engineering and Technology 2013 12:2171-2180
Received: December 07, 2012 | Accepted: January 11, 2013 | Published: July 30, 2013
Abstract
Identifying factors having influential impact on automobile demand estimation has become a primary concern for automobile industry. There has been substantial development modeling for automobile demand estimation. The Regression, Time series, CHAID and RBF neural network modeling types are proposed. In this study, automobile demand is classified into three classes. The first issue to be addressed by this study is to selecting the most appropriate modeling types in each class by comparing the actual demand and estimated demand in terms of Root-Mean-Square-Error (RMSE). Results indicate that modeling can be different for different classes of automobile demand. The second aim of this study is to identify the significant indicators for estimating automobile demand in each class of Iranian market.
Keywords:
CHAID method, RBF neural network, regression method, RMSE, time series method,
Competing interests
The authors have no competing interests.
Open Access Policy
This article is distributed under the terms of the Creative Commons Attribution 4.0 International License (http://creativecommons.org/licenses/by/4.0/), which permits unrestricted use, distribution, and reproduction in any medium, provided you give appropriate credit to the original author(s) and the source, provide a link to the Creative Commons license, and indicate if changes were made.
Copyright
The authors have no competing interests.
|
|
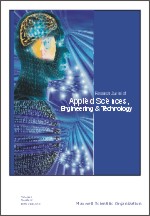 |
ISSN (Online): 2040-7467
ISSN (Print): 2040-7459 |
 |
Information |
|
|
|
Sales & Services |
|
|
|