Research Article | OPEN ACCESS
Linear Projective Non-Negative Matrix Factorization
1, 2, 3Lirui Hu, 1, 3Jianguo Wu and 1, 3Lei Wang
1Key Laboratory of Intelligent Computing and Signal Processing of Ministry of Education,
Anhui University, Hefei 230039, China
2School of Computer Science and Technology, Nantong University, Nantong 226019, China
3School of Computer Science and Technology, Anhui University, Hefei 230039, China
Research Journal of Applied Sciences, Engineering and Technology 2013 9:1626-1631
Received: December 23, 2012 | Accepted: January 25, 2013 | Published: July 15, 2013
Abstract
In order to solve the problem that the basis matrix is usually not very sparse in Non-Negative Matrix Factorization (NMF), a method, called Linear Projective Non-Negative Matrix Factorization (LP-NMF), is proposed. In LP-NMF, from projection and linear transformation angle, an objective function of Frobenius norm is defined. The Taylor series expansion is used. An iterative algorithm for basis matrix and linear transformation matrix is derived and a proof of algorithm convergence is provided. Experimental results show that the algorithm is convergent; relative to Non-negative Matrix Factorization (NMF), the orthogonality and the sparseness of the basis matrix are better; in face recognition, there is higher recognition accuracy. The method for LP-NMF is effective.
Keywords:
Face recognition, linear transformation, non-negative matrix factorization, projective,
Competing interests
The authors have no competing interests.
Open Access Policy
This article is distributed under the terms of the Creative Commons Attribution 4.0 International License (http://creativecommons.org/licenses/by/4.0/), which permits unrestricted use, distribution, and reproduction in any medium, provided you give appropriate credit to the original author(s) and the source, provide a link to the Creative Commons license, and indicate if changes were made.
Copyright
The authors have no competing interests.
|
|
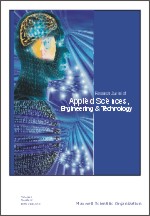 |
ISSN (Online): 2040-7467
ISSN (Print): 2040-7459 |
 |
Information |
|
|
|
Sales & Services |
|
|
|