Research Article | OPEN ACCESS
A BP Neural Network Based on Improved Particle Swarm Optimization and its Application in Reliability Forecasting
1Heqing Li and 2Qing Tan
1School of Mechanical and Electrical Engineering, Central
South University, Changsha 410083, China
2School of Automobile and Mechanical Engineering, Changsha University of Science of Technology, Changsha 410076, China
Research Journal of Applied Sciences, Engineering and Technology 2013 7:1246-1251
Received: October 30, 2012 | Accepted: December 21, 2012 | Published: July 05, 2013
Abstract
The basic Particle Swarm Optimization (PSO) algorithm and its principle have been introduced, the Particle Swarm Optimization has low accelerate speed and can be easy to fall into local extreme value, so the Particle Swarm Optimization based on the improved inertia weight is presented. This method means using nonlinear decreasing weight factor to change the fundamental ways of PSO. To allow full play to the approximation capability of the function of BP neural network and overcome the main shortcomings of its liability to fall into local extreme value and the study proposed a concept of applying improved PSO algorithm and BP network jointly to optimize the original weight and threshold value of network and incorporating the improved PSO algorithm into BP network to establish a improved PSO-BP network system. This method improves convergence speed and the ability to search optimal value. We apply the improved particle swarm algorithm to reliability prediction. Compared with the traditional BP method, this kind of algorithm can minimize errors and improve convergence speed at the same time.
Keywords:
BP improvement, neural network, reliability prediction, the improved PSO,
Competing interests
The authors have no competing interests.
Open Access Policy
This article is distributed under the terms of the Creative Commons Attribution 4.0 International License (http://creativecommons.org/licenses/by/4.0/), which permits unrestricted use, distribution, and reproduction in any medium, provided you give appropriate credit to the original author(s) and the source, provide a link to the Creative Commons license, and indicate if changes were made.
Copyright
The authors have no competing interests.
|
|
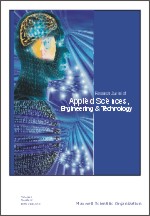 |
ISSN (Online): 2040-7467
ISSN (Print): 2040-7459 |
 |
Information |
|
|
|
Sales & Services |
|
|
|