Research Article | OPEN ACCESS
Optimization of PID Controller for Brushless DC Motor by using Bio-inspired Algorithms
1Sanjay Kr. Singh, 2Nitish Katal and 3S.G. Modani
1Anand International College of Engineering, Jaipur, Rajasthan, India
2Department of Electronics and Communication Engineering,
Amity University, Rajasthan, India
3Malaviya National Institute of Technology, Jaipur, Rajasthan, India
Research Journal of Applied Sciences, Engineering and Technology 2014 7:1302-1308
Received: April 16, 2013 | Accepted: May 21, 2013 | Published: February 20, 2014
Abstract
This study presents the use and comparison of various bio-inspired algorithms for optimizing the response of a PID controller for a Brushless DC Motor in contrast to the conventional methods of tuning. For the optimization of the PID controllers Genetic Algorithm, Multi-objective Genetic Algorithm and Simulated Annealing have been used. PID controller tuning with soft-computing algorithms comprises of obtaining the best possible outcome for the three PID parameters for improving the steady state characteristics and performance indices like overshoot percentage, rise time and settling time. For the calculation and simulation of the results the Brushless DC Motor model, Maxon EC 45 flat ф 45 mm with Hall Sensors Motor has been used. The results obtained the optimization using Genetic Algorithms, Multi-objective Genetic Algorithm and Simulated Annealing is compared with the ones derived from the Ziegler-Nichols method and the MATLAB SISO Tool. And it is observed that comparatively better results are obtained by optimization using Simulated Annealing offering better steady state response.
Keywords:
Brushless DC motor, controller tuning, genetic algorithm, PID controllers, PID optimization, simulated annealing, ziegler nichols,
References
-
Åström, K.J., P. Albertos and J. Quevedo, 2001. PID control. Control Eng. Pract., 9: 159-1161.
CrossRef
-
Berrsimas, D., 1993. Tsitsiklis: Simulated annealing. Stat. Sci., 8(1): 10-15.
CrossRef
-
Deb, K., 2001. Multi-Objective Optimization Using Evolutionary Algorithms. John Wiley and Sons, Ltd., Chichester.
-
Goodwin, G.C., S.F. Graebe and M.E. Salgado, 2001. Control System Design. Prentice Hall Inc., New Jersey.
-
Jean-Pierre, C., 2004. Process Control: Theory and Applications. Springer, London, pp: 752.
CrossRef
-
Katal, N., S.K. Singh and M. Agrawal, 2012. Optimizing response of PID controller for servo DC motor by genetic algorithm. Int. J. Appl. Eng. Res., 7(11).
-
Krause, P.C., O. Wasynozuk and S.D. Sudhoff, 2002. Analysis of Electric Machinery and Drive Systems. 2nd Edn., IEEE Press, New York.
CrossRef
-
Norman, S.N., 2003. Control Systems Engineering, JustAsk! Control Solutions Companion. 4th Edn., Wiley, Hoboken, N.J, pp: 96.
-
Padmaraja, Y., 2003. Brushless DC Motor Fundamentals. Microchip Technology Incorporated.
Direct Link
-
Sabudin, E.N.B., 2012. Development of position tracking of BLDC motor using adaptive fuzzy logic controller. M.A. Thesis. Faculty of Electrical and Electronic Engineering, Universiti Tun Hussein Onn, Malaysia.
-
Ziegler, J.G. and N.B. Nichols, 1942. Optimum settings for automatic controllers. Trans. ASME, 64: 759-768.
Competing interests
The authors have no competing interests.
Open Access Policy
This article is distributed under the terms of the Creative Commons Attribution 4.0 International License (http://creativecommons.org/licenses/by/4.0/), which permits unrestricted use, distribution, and reproduction in any medium, provided you give appropriate credit to the original author(s) and the source, provide a link to the Creative Commons license, and indicate if changes were made.
Copyright
The authors have no competing interests.
|
|
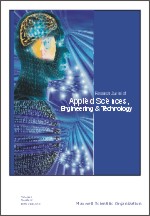 |
ISSN (Online): 2040-7467
ISSN (Print): 2040-7459 |
 |
Information |
|
|
|
Sales & Services |
|
|
|