Research Article | OPEN ACCESS
A Gray Texture Classification Using Wavelet and Curvelet Coefficients
1M. Santhanalakshmi and 2K. Nirmala
1Department of Computer Application, Manonmaniam Sundranar University, Tamilnadu, India
2Quaid-e-Millath Government College for Women, Tamilnadu, India
Research Journal of Applied Sciences, Engineering and Technology 2014 24:5258-5263
Received: February 28,2014 | Accepted: April 08, 2014 | Published: June 25, 2014
Abstract
This study presents a framework for gray texture classification based on wavelet and curvelet features. The two main frequency domain transformations Discrete Wavelet Transform (DWT) and Discrete Curvelet Transform (DCT) are analyzed. The features are extracted from the DWT and DCT decomposed image separately and their performances are evaluated independently. The performance metric used to analyze the system is classification accuracy. The standard benchmark database, Brodatz texture images are used for this study. The results show that, the curvelet based features provides better accuracy than wavelet based features.
Keywords:
Brodatz album, curvelet transform, nearest neighbor classifier, texture classification, wavelet transform,
References
-
Candè€s, E.J., L. Demanet, D.L. Donoho and L. Ying, 2005. Fast discrete curvelet transforms. Multiscale Model. Sim., 5: 861-899.
CrossRef -
Dong, Y. and J. Ma, 2011. Wavelet-based image texture classification using local energy histograms. IEEE Signal Proc. Let., 18(4): 247-250.
CrossRef -
Dong, Y. and J. Ma, 2012. Bayesian texture classification based on contourlet transform and BYY harmony learning of poisson mixtures. IEEE T. Image Process., 21(3): 909-918.
CrossRef PMid:21947521 -
Donoho, D.L. and M.R. Duncan, 1999. Digital curvelet transform: Strategy, implementation and experiments. Technical Report, Stanford University, Stanford, Calif.
PMid:10051554 PMCid:PMC26696 -
Guo, Z., D. Zhang and D. Zhang, 2010. A completed modeling of local binary pattern operator for texture classification. IEEE T. Image Process., 19(6): 1657-1663.
CrossRef PMid:20215079 -
He, J., H. Ji and X. Yang, 2013. Rotation invariant texture descriptor using local shearlet-based energy histograms. IEEE Signal Proc. Let., 20(9): 905-908.
CrossRef -
Ji, H., X. Yang, H. Ling and Y. Xu, 2013. Wavelet domain multi-fractal analysis for static and dynamic texture classification. IEEE T. Image Process., 22(1): 286-299.
CrossRef PMid:22910109 -
Khellah, F.M., 2011. Texture classification using dominant neighborhood structure. IEEE T. Image Process., 20(11): 3270-3279.
CrossRef PMid:21511565 -
Lategahn, H., S. Gross, T. Stehle and T. Aach, 2010. Texture classification by modeling joint distributions of local patterns with Gaussian mixtures. IEEE T. Image Process., 19(6): 1548-1557.
CrossRef PMid:20129862 -
Liu, L. and P.W. Fieguth, 2012. Texture classification from random features. IEEE T. Pattern Anal., 34(3): 574-586.
CrossRef PMid:21768653 -
Maani, R., S. Kalra and Y. Yang, 2013. Rotation invariant local frequency descriptors for texture classification. IEEE T. Image Process., 22(6): 2409-2419.
CrossRef PMid:23475362 -
Mallat, S.G., 1989. A theory for multi resolution signal decomposition: The wavelet representation. IEEE T. Pattern Anal., 11(7): 674-693.
CrossRef -
Riaz, F., A. Hassan, S. Rehman and U. Qamar, 2013. Texture classification using rotation-and scale-invariant Gabor texture features. IEEE Signal Proc. Let., 20(6): 607-610.
CrossRef -
Starck, J.L., E. Candes and D.L. Donoho, 2002. The curvelet transform for image denoising, IEEE T. Image Process., 11(6): 670-684.
CrossRef PMid:18244665 -
Zhang, J., J. Liang and H. Zhao, 2013. Local energy pattern for texture classification using self-adaptive quantization thresholds. IEEE T. Image Process., 22: 31-42.
CrossRef PMid:22910113 -
Zhao, Y., D.S. Huang and W. Jia, 2012. Completed local binary count for rotation invariant texture classification. IEEE T. Image Process., 21(10): 4492-4497.
CrossRef PMid:22711773
Competing interests
The authors have no competing interests.
Open Access Policy
This article is distributed under the terms of the Creative Commons Attribution 4.0 International License (http://creativecommons.org/licenses/by/4.0/), which permits unrestricted use, distribution, and reproduction in any medium, provided you give appropriate credit to the original author(s) and the source, provide a link to the Creative Commons license, and indicate if changes were made.
Copyright
The authors have no competing interests.
|
|
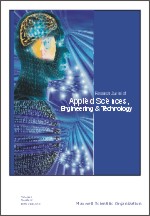 |
ISSN (Online): 2040-7467
ISSN (Print): 2040-7459 |
 |
Information |
|
|
|
Sales & Services |
|
|
|