Research Article | OPEN ACCESS
An Efficient Fingerprint Based Gender Classification System Using Dominant Un-decimated Wavelet Coefficients
1D. Gnana Rajesh and 2M. Punithavalli
1Department of Computer Science, Manonmaniam Sundaranar University, Tamilnadu, India
2Department of the Computer Science, Sri Ramakrishna College of Arts and Science for Women, Coimbatore, India
Research Journal of Applied Sciences, Engineering and Technology 2014 10:1259-1265
Received: June 11, 2014 | Accepted: July 19, 2014 | Published: September 15, 2014
Abstract
Gender classification is the major and challenging task in the field of forensic anthropology which minimizes the list of suspects search. The existing systems use the availability of bones, teeth and other identifiable body parts having physical features that allow gender and age estimation by conventional methods. The different biometrics traits such as face, gait, iris, speech and fingerprint are used to identify the gender and age. Among the biometrics, fingerprint is most commonly available in any crime scene. In this study, an efficient algorithm to identify the gender of a given fingerprint into male or female is proposed. The two most efficient techniques are utilized to enhance the performance of the gender classification system. As the first step, Un-decimated Wavelet Transform (UWT) is employed to extract the features from the fingerprints by applying ranking. Secondly, Gaussian Mixture Models (GMMs) technique is used as classifier for the process of gender classification. The proposed system is carried out with the database of 180 persons finger prints of all fingers in which 80 are female and 100 are male. The results show the satisfactory classification accuracy of over 90%.
Keywords:
Gaussian mixture model, gender classification, stationary wavelet transform, wavelet transform,
References
-
Arun, K.S. and K.S. Sarath, 2011. A machine learning approach for fingerprint based gender identification. Proceeding of IEEE Recent Advances in Intelligent Computational Systems (RAICS, 2011), pp: 163-167.
CrossRef -
Badawi, A., M. Mahfouz, R. Tadross and R. Jantz, 2006. Fingerprint-based gender classification. Proceeding of the International Conference on Image Processing, Computer Vision and Pattern Recognition, pp: 41-46.
-
Bharti, P. and C.S. Lamba, 2014. DWT-neural network based gender classification. Int. J. Dig. Appl. Contemp. Res., 2(8).
-
Bishop, C.M., 2006. Pattern Recognition and Machine Learning. Chapter 9, Springer, New York, 1: 435.
-
Gnanasivam, P. and S. Muttan, 2012. Fingerprint gender classification using wavelet transform and singular value decomposition. Int. J. Comput. Sci. Issues, 9(2).
-
Gornale, S.S., C.D. Geetha and R. Kruthi, 2013. Analysis of fingerprint image for gender classification using spatial and frequency domain analysis. Amer. Int. J. Res. Sci. Technol. Eng. Math., 1(1): 46-50.
-
Gupta, S. and A.P. Rao, 2014. Fingerprint based gender classification using discrete wavelet transform and artificial neural network. Int. J. Comput. Sci. Mob. Comput., 3(4): 1289-1296.
-
Holschneider, M., R. Kronland-Martinet, J. Morlet and P. Tchamitchian, 1990. A real-time Algorithm for Signal Analysis with the Help of the Wavelet Transform. In: Combes, J.M. et al., (Eds.), Wavelets. Springer-Berlin, Heidelberg, pp: 286-297.
CrossRef -
Kaur, R. and S.G. Mazumdar, 2012. Fingerprint based gender identification using frequency domain analysis. Int. J. Adv. Eng. Technol., 3(1): 295-299.
-
Mallat, S., 1998. A Wavelet Tour of Signal Processing. Academic Press, New York.
-
Ponnarasi, S.S. and M. Rajaram, 2012. Gender classification system derived from fingerprint minutiae extraction. Proceeding of IJCA International Conference on Recent Trends in Computational Methods, Communication and Controls (ICON3C, 2012), pp: 1-6.
-
Purohit, R.V., S.A. Imam and M.T. Beg, 2011. Recognizing gender with fingerprints. Int. J. Adv. Eng. Technol., 2(4): 239-241.
-
Shensa, M., 1992. The Discrete wavelet transform: Wedding the a trous and Mallat algorithms. IEEE T. Signal Proces., 40(10): 2464-2482.
CrossRef -
Tom, R.J., T. Arulkumaran and M.E. Scholar, 2013. Fingerprint based gender classification using 2d discrete wavelet transforms and principal component analysis. Int. J. Eng. Trends Technol., 4(2): 199-203.
-
Wadhwa, R., M. Kaur and K.V.P. Singh, 2013. Age and gender determination from finger prints using RVA and DCT coefficients. IOSR J. Eng. (IOSRJEN), 3(8): 5-9.
CrossRef -
Wang, J.F., C.L. Lin, Y.H. Chang, M.L. Nagurka, C.W. Yen and C. Yeh, 2008. Gender determination using fingertip features. Internet J. Med. Update, 3(2): 22-28.
Competing interests
The authors have no competing interests.
Open Access Policy
This article is distributed under the terms of the Creative Commons Attribution 4.0 International License (http://creativecommons.org/licenses/by/4.0/), which permits unrestricted use, distribution, and reproduction in any medium, provided you give appropriate credit to the original author(s) and the source, provide a link to the Creative Commons license, and indicate if changes were made.
Copyright
The authors have no competing interests.
|
|
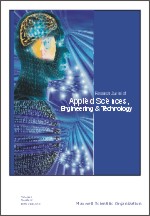 |
ISSN (Online): 2040-7467
ISSN (Print): 2040-7459 |
 |
Information |
|
|
|
Sales & Services |
|
|
|