Research Article | OPEN ACCESS
Sparse Space Replica Based Image Reconstruction via Cartesian and Spiral Sampling Strategies
1K.L. Nisha, 2B. Shaji and 3N.R. Rammohan
1Department of Electronics and Communication and Engineering, Arunachala College of
Engineering for Woman
2Department of Information Technology, Marthandam College of Engineering and Technology
3Department of Computer Science and Engineering, Sun College of Engineering and Technology, Nagercoil, Tamilnadu, India
Research Journal of Applied Sciences, Engineering and Technology 2014 11:1340-1349
Received: May 31, 2014 | Accepted: July 13, 2014 | Published: September 20, 2014
Abstract
In this study, a replica based image reconstruction is designed to provide high-quality reconstructions from very sparse space data. The problem of reconstructing an image from its unequal frequency samples take place in many applications. Images are observed on a spherical manifold, where one seeks to get an improved unidentified image from linear capacity, which is noisy, imperfect through a convolution procedure. Existing framework for Total Variation (TV) inpainting on the sphere includes fast methods to render the inpainting problem computationally practicable at high-resolution. In recent times, a new sampling theorem on the sphere developed, reduces the necessary number of samples by a feature of two for equiangular sampling schemes but the image that is not extremely sparse in its gradient. Total Variation (TV) inpainting fails to recover signals in the spatial domain directly with improved dimensionality signal. The regularization behavior is explained by using the theory of Lagrangian multiplier but space limitation fails to discover effective connection. To overcome these issues, Replica based Image Reconstruction (RIR) is developed in this study. RIR presents reconstruction results using both Cartesian and spiral sampling strategies using data simulated from a real acquisition to improved dimensionality signal in the spatial domain directly. RIR combined with the Global Reconstruction Constraint to remove the noisy imperfect area and highly sparse in its gradient. The proposed RIR method leads to significant improvements in SNR with very sparse space for effective analytical connection result. Moreover, the gain in SNR is traded for fewer space samples. Experimental evaluation is performed on the fMRI Data Set for Visual Image Reconstruction. RIR method performance is compared against the exiting TV framework in terms of execution time, Noisy area in SNR, accuracy rate, computational complexity, mean relative error and image dimensionality enhancement.
Keywords:
Cartesian , dimensionality signal , frequency samples , global constraint , replica based image reconstruction , sparse data , spiral sampling strategies , total variation method,
References
-
Afonso, M.V., J.M. Bioucas-Dias and M.A.T. Figueired, 2011. An augmented lagrangian approach to the constrained optimization formulation of imaging inverse problems. IEEE T. Image Process., 20(3): 681-695.
CrossRef PMid:20840899 -
Argyriou, V., 2011. Sub-hexagonal phase correlation for motion estimation. IEEE T. Image Process., 20(1): 110-120.
CrossRef PMid:20624707 -
Averbuch, A., G. Lifschitz and Y. Shkolnisky, 2011. Accelerating x-ray data collection using pyramid beam ray casting geometries. IEEE T. Image Process., 20(2): 523-533.
CrossRef PMid:20693110 -
Babacan, S.D., R. Molina and A.K. Katsaggelos, 2011. Variational bayesian super resolution. IEEE T. Image Process., 20(4):984-999.
CrossRef PMid:20876021 -
Chen, B., H. Shu, H. Zhang, G. Coatrieux, L. Luo and J.L. Coatrieux, 2011. Combined invariants to similarity transformation and to blur using orthogonal Zernike moments. IEEE T. Image Process., 20(2): 345-360.
CrossRef PMid:20679028 PMCid:PMC3286441 -
Chouzenoux, E., J. Idier and S. Moussaoui, 2011. A majorize-minimize strategy for subspace optimization applied to image restoration. IEEE T. Image Process., 20(6): 1517-1528.
CrossRef PMid:21193375 -
Gajjar, P.P. and M.V. Joshi, 2010. New learning based super-resolution: Use of DWT and IGMRF prior. IEEE T. Image Process., 19(5): 1201-1213.
CrossRef PMid:20106738 -
Giannoula, A., 2011. Classification-based adaptive filtering for multiframe blind image restoration. IEEE T. Image Process., 20(2): 382-390.
CrossRef PMid:20693109 -
Hu, Y., K.M. Lam, G. Qiu and T. Shen, 2011. From local pixel structure to global image super-resolution: A new face hallucination framework. IEEE T. Image Process., 20(2): 433-445.
CrossRef PMid:20693112 -
Hua, G. and O.G. Guleryuz, 2011. Spatial Sparsity-Induced Prediction (SIP) for images and video: A simple way to reject structured interference. IEEE T. Image Process., 20(4): 889-909.
CrossRef PMid:20923739 -
Katsuki, T., A. Torii and M. Inoue, 2012. Posterior mean super-resolution with a causal Gaussian Markov random field prior. IEEE T. Image Process., 21(7): 3182-3193.
CrossRef PMid:22389146 -
Koo, H.I. and N.I. Cho, 2011. Design of interchannel mrf model for probabilistic multichannel image processing. IEEE T. Image Process., 20(3): 601-611.
CrossRef PMid:20875973 -
Lefkimmiatis, S., A. Bourquard and M. Unser, 2011. Hessian-based norm regularization for image restoration with biomedical applications. IEEE T. Image Process., 21(3): 983-995.
CrossRef PMid:21937351 -
Li, X., 2011. Fine-granularity and spatially-adaptive regularization for projection-based image deblurring. IEEE T. Image Process., 20(4): 971-983.
CrossRef PMid:20876018 -
Liao, H. and M.K. Ng, 2011. Blind deconvolution using generalized cross-validation approach to regularization parameter estimation. IEEE T. Image Process., 20(3): 670-680.
CrossRef PMid:20833603 -
McEwen, J.D., G. Puy, J.P. Thiran, P. Vandergheynst, D. Van De Ville and Y. Wiaux, 2013. Sparse image reconstruction on the sphere: Implications of a new sampling theorem. IEEE T. Image Process., 22(6).
-
Montefusco, L.B., D. Lazzaro and S. Papi, 2011. Fast sparse image reconstruction using adaptive nonlinear filtering. IEEE T. Image Process., 20(2): 534-544.
CrossRef PMid:20679029 -
Shaked, E. and O. Michailovich, 2011. Iterative shrinkage approach to restoration of optical imagery. IEEE T. Image Process., 20(2): 405-416.
CrossRef PMid:20801739 -
Shi, G., D. Gao, X. Song, X. Xie, X. Chen and D. Liu, 2011. High-resolution imaging via moving random exposure and its simulation. IEEE T. Image Process., 20(1): 276-282.
CrossRef PMid:20529739 -
Woolfe, F., M. Gerdes, M. Bello, X. Tao and A. Can, 2011. Autofluorescence removal by non-negative matrix factorization. IEEE T. Image Process., 20(4): 1085-1093.
CrossRef PMid:20889433
Competing interests
The authors have no competing interests.
Open Access Policy
This article is distributed under the terms of the Creative Commons Attribution 4.0 International License (http://creativecommons.org/licenses/by/4.0/), which permits unrestricted use, distribution, and reproduction in any medium, provided you give appropriate credit to the original author(s) and the source, provide a link to the Creative Commons license, and indicate if changes were made.
Copyright
The authors have no competing interests.
|
|
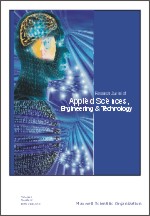 |
ISSN (Online): 2040-7467
ISSN (Print): 2040-7459 |
 |
Information |
|
|
|
Sales & Services |
|
|
|