Research Article | OPEN ACCESS
Email Phishing Detection System Using Neural Network
Amina A. Abdullah, Loay E. George and Imad J. Mohammed
College of Science, University of Baghdad, Iraq
Research Journal of Information Technology 2015 3:39-43
Received: May 17, 2015 | Accepted: July 10, 2015 | Published: August 05, 2015
Abstract
One of the internet based identity thefts is called phishing. Email phishing is a way that phishers trick the user to give information. The increasing of the phish attack in the resent years cause a lot of problems; credit card number, user name, password were stolen due to the phish attack. Due to this attack people lose their money, personal information and trust in online business also the attack effects on the companies' reputation. Many companies were losing money up to millions of dollars. In this study a new stem that can quickly detect phishing emails with low false positive rate is introduced. A set of features is proposed to test each coming emails to identify whether it is phish email or not. A feed forward neural network with back propagation training algorithm was adopted to categorize the email samples into phish or ham category. First a set of extracted features vectors from each email, each vector consists of 17 features, had been used to make the categorization decision. Then, a set of smaller feature vectors, each consists of only the 12 best features, was used for classification tests. The results achieved in this study are 99.91 (for 17 features) and 99.95for (12 best features).
Keywords:
Email phishing, email threats, machine learning (neural network), phishing detection,
References
-
Aaron, G., R. Rasmussen and A. Routt, 2014. Global Phishing Survey: Trends and Domain Name Use in 1H2014. Anti Phishing Working Group (APWG), Lexington.
-
Aaron, G. and R. Rasmussen, 2015. Global Phishing Survey: Trends and Domain Name Use in 2H2014. Anti Phishing Working Group (APWG), http://www.apwg.org ? info@apwg.org.
Direct Link -
Aggarwaly, A., A. Rajadesingan and P. Kumaraguru, 2012. PhishAri: Automatic Realtime Phishing Detection on Twitter. Proceedings of the IEEE eCrime Researchers Summit (eCrime), Las Croabas, pp: 1-12.
-
Almomani, A., B.B. Gupta, S. Atawneh, A. Meulenberg and E. Almomani, 2013. A survey of phishing email filtering techniques. IEEE Commun. Surv. Tutorials, 15(4): 2070-2090.
CrossRef -
Chandrasekaran, M., R. Chinchani and S. Upadhyaya, 2006. PHONEY: Mimicking user response to detect phishing attacks. Proceedings of the IEEE International Symposium on a World of Wireless Mobile and Multimedia Network (WoWMoM), Buffalo-Niagara Falls, NY, USA, pp: 672-676.
CrossRef -
Fette, I., N. Sadeh and A. Tomasic, 2007. Learning detection phishing emails. Proceedings of the International World Wide Web Conference Committee (IW3C2), Banff, Alberta, Canada, pp: 649-656.
-
Ghazhie, N. and L.E. George, 2013. Methodologies to Detect Phishing Emails. Published by Scholars' Press. Retrieved from: http://dl.acm.org/citation.cfm?id=2613502.
Direct Link -
Kavada, A., 2007. The European Social Forum and the Internet: A Case Study of Communication Networks and Collective Action. PHD Thesis, University of Westminster, London, United Kingdom.
-
Khonji, M., Y. Iraqi and A. Jones, 2011. Lexical URL analysis for discriminating phishing and legitimate e-mail messages. Proceedings of the IEEE 6th International Conference Internet Technology and Secured Transactions (ICITST), Abu Dhabi, United Arab Emirates, pp: 422-427.
-
Khonji, M., Y. Iraqi and A. Jones, 2012. Enhancing phishing e-mail classifiers: A lexical URL analysis approach. Int. J. Inform. Secur. Res., 2(1/2): 236-245.
-
Tally, G., R. Thomas and T.V. Vleck, 2004. Anti-Phishing: Best Practices for Institutions and Consumers. White Paper, McAfee Inc.
-
Vaishnaw, N. and S.R. Tandan, 2015. Development of anti-phishing model for classification of phishing e-mail. IEEE Int. J. Adv. Res. Comp. Commun. Eng., 4(6): 39-45.
Competing interests
The authors have no competing interests.
Open Access Policy
This article is distributed under the terms of the Creative Commons Attribution 4.0 International License (http://creativecommons.org/licenses/by/4.0/), which permits unrestricted use, distribution, and reproduction in any medium, provided you give appropriate credit to the original author(s) and the source, provide a link to the Creative Commons license, and indicate if changes were made.
Copyright
The authors have no competing interests.
|
|
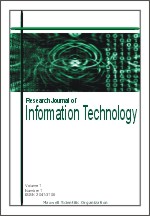 |
ISSN (Online): 2041-3114
ISSN (Print): 2041-3106 |
 |
Information |
|
|
|
Sales & Services |
|
|
|