Research Article | OPEN ACCESS
Anomalies Calculation and Detection in Fuel Expense through Data Mining
Kaleem Habib and Arif Iqbal Umar
Department of Information Technology, Hazara University, Mansehra, Pakistan
Research Journal of Information Technology 2015 4:44-50
Received: September 9, 2015 | Accepted: October 11, 2015 | Published: August 05, 2015
Abstract
In organizations having large vehicle fleet a reasonable portion of the fuel budget is misused by malpractices of drivers and fuel providers. An optimal usage of this fuel amount could be of big advantages to the organization. We proposed a novel anomaly %age calculation algorithm to determine the misuse of the fuel of the vehicles. This algorithm will reduce the efforts for anomaly detection in clustering process. The results reflect that this algorithm could be used to implement an effective check on the misuse of the fuel in big organizations with less effort.
Keywords:
Anomaly, data mining, fraud, fuel,
References
-
Annie, G., 2012. Anomaly detection based on machine learning: Dimensionality reduction using PCA and classification using SVM. Int. J. Comp. Appl., 47(21): 5-8.
-
Ashoor, A.S. and S. Gore, 2011. Importance of Intrusion Detection System (IDS). Int. J. Scient. Eng. Res., 2(1): 1-4.
-
Basha, M.A., 2012. A simplified approach to agent based efficient anomaly intrusion detection in ad-hoc networks using honey tokens. Int. J. Eng. Res. Appli., 2(3): 2242-2247.
-
Janakiraman, S. and V. Vasudevan, 2009. An intelligent distributed intrusion detection system using genetic algorithm. J. Converg. Inform. Technol., 4(1): 70-76.
CrossRef -
Pari, S.N. and D. Sridharan, 2011. A performance comparison and evaluation of analysing node misbehaviour in MANET using intrusion detection system. Int. J. Comp. Sci. Emer. Technol., 1(1): 35-40.
-
Ramos-Morillo, H., I. Lorenzo-Fonseca, F. Mora-Gimeno, D. Marcos-Jorquera and J.A. Gil-MartiŽnez-Abarca, 2010. Network intrusion detection system embedded on a smart sensor. IEEE Trans. Indus. Elec., 58(3): 722-732.
-
Ringberg, H., A. Soule, J. Rexford and C. Diot, 2007. Sensitivity of PCA for traffic anomaly detection. Proceedings of the ACM SIGMETRICS International Conference on Measurement and Modeling of Computer Systems, San Diego, California, USA.
CrossRef -
Shah, B. and B.H. Trivedi, 2012. Artificial neural network based intrusion detection system: A survey. Int. J. Comp. Appl., 39(6): 13-18.
CrossRef -
Snelding, K., 2010. Audit of East Service Center Employee Fuel Theft, Report.
-
Thiprungsri, S., 2010. Cluster analysis for anomaly detection in accounting data. Proceedings of the 19th Annual Strategic and Emerging Technologies Research Workshop, San Francisco, California, USA.
-
Thottan, M. and J. Chuanyi, 2003. Anomaly detection in IP networks. IEEE Trans. Signal Proc., 51(8): 2191-2204.
CrossRef
Competing interests
The authors have no competing interests.
Open Access Policy
This article is distributed under the terms of the Creative Commons Attribution 4.0 International License (http://creativecommons.org/licenses/by/4.0/), which permits unrestricted use, distribution, and reproduction in any medium, provided you give appropriate credit to the original author(s) and the source, provide a link to the Creative Commons license, and indicate if changes were made.
Copyright
The authors have no competing interests.
|
|
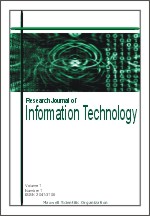 |
ISSN (Online): 2041-3114
ISSN (Print): 2041-3106 |
 |
Information |
|
|
|
Sales & Services |
|
|
|