Research Article | OPEN ACCESS
Blind Audio Source Separation with Sparse Nonnegative Matrix Factorization
Abd Majid Darsono, N.Z. Haron, Shakir Saat, M.M. Ibrahim and N.A. Manap
Faculty of Electronics and Computer Engineering, Universiti Teknikal Malaysia Melaka Hang Tuah Jaya, 76100 Durian Tunggal, Melaka, Malaysia
Research Journal of Applied Sciences, Engineering and Technology 2014 23:5015-5020
Received: February 14, 2014 | Accepted: April 17, 2014 | Published: June 20, 2014
Abstract
In this study, a new technique in source separation using Two-Dimensional Nonnegative Matrix Factorization (NMF2D) with the Beta-divergence is proposed. The Time-Frequency (TF) profile of each source is modeled as two-dimensional convolution of the temporal code and the spectral basis. In addition, adaptive sparsity constraint was imposed to reduce the ambiguity and provide uniqueness to the solution. The proposed model used Beta-divergence as a cost function and updated by maximizing the joint probability of the mixing spectral basis and temporal codes using the multiplicative update rules. Experimental tests have been conducted in audio application to blindly separate the source in musical mixture. Results have shown the effectiveness of the algorithm in separating the audio sources from single channel mixture.
Keywords:
Beta divergence, blind audio source separation, machine learning, nonnegative matrix factorization,
References
-
Biciu, I., N. Nikolaidis and I. Pitas, 2007. Nonnegative matrix factorization in polynomial feature space. IEEE T. Neural Networ., 19: 1090-1100.
CrossRef PMid:18541506
-
Fevotte, C., R. Gribonval and E. Vincent, 2005. BSS EVAL toolbox user guide. IRISA Technical Report 1706, Rennes, France.
-
Fevotte, C., N. Bertin and J.L. Durrieu, 2009. Nonnegative matrix factorization with the Itakura-Saito divergence with application to music analysis. Neural Comput., 21: 793-830.
CrossRef PMid:18785855
-
FitzGerald, D., 2004. Automatic drum transcription and source separation. Ph.D. Thesis, Dublin Institute of Technology, Dublin, Ireland.
-
Kompass, R., 2005. Generalized divergence measure for non-negative matrix factorization. Proceeding of the Neuroinformatics Workshop. Torun, Poland.
-
Lee, C. and H. Seung, 1999. Learning the parts of objects by nonnegative matrix factorisation. Nature, 401(6755): 788-791.
CrossRef PMid:10548103
-
Morup, M. and M.N. Schmidt, 2006. Sparse nonnegative matrix factor 2-D deconvolution. Techical Report, Technical University of Denmark, Copenhagen, Denmark.
-
Wang, D.L., 2005. On Ideal Binary Mask as the Computational Goal of Auditory Scene Analysis. In: Diventi, P. (Ed.), Speech Separation by Humans and Machines. Kluwer, Norwell, MA, pp: 181-197.
CrossRef
-
Xie, S., Z. Yang and Y. Fu, 2008. Nonnegative matrix factorization applied to nonlinear speech and image cryptosystems. IEEE T. Circuits-I, 55(8): 2356-2367.
CrossRef
Competing interests
The authors have no competing interests.
Open Access Policy
This article is distributed under the terms of the Creative Commons Attribution 4.0 International License (http://creativecommons.org/licenses/by/4.0/), which permits unrestricted use, distribution, and reproduction in any medium, provided you give appropriate credit to the original author(s) and the source, provide a link to the Creative Commons license, and indicate if changes were made.
Copyright
The authors have no competing interests.
|
|
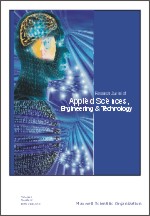 |
ISSN (Online): 2040-7467
ISSN (Print): 2040-7459 |
 |
Information |
|
|
|
Sales & Services |
|
|
|