Research Article | OPEN ACCESS
A Neural Network Predictive Model for Welded Marine Pipeline Internal Corrosion
1Abdulwahab A. Alnaqi, 2N.S. Reddy, 1Khaled Alawadhi, 1Ahmad E. Murad and 1Hasan A. Mulla Ali
1Departament of Automotive and Marine Engineering Technology, College of Technological Studies-PAAET, Kuwait
2School of Material Science and Engineering, Gyeongsang National University, Jinju, 660-701, Republic of Korea
Research Journal of Applied Sciences, Engineering and Technology 2016 7:585-592
Received: May 13, 2016 | Accepted: July 14, 2016 | Published: October 05, 2016
Abstract
In spite of the huge success of computational chemistry in corrosion studies, most of the ongoing research on the inhibition of preferential weldcorrosion is restricted to laboratory work. In the present study, a nondeterministic artificial intelligence model is proposed, the aim being to more accurately predict the occurrence of corrosion in the Heat Affected Zone (HAZ), which is most exposed to corrosion risk. The prediction of corrosion rates has become an important challenge for the engineering community. For industry, one of the more important aspects of corrosion is the HAZ for welded carbon steel in CO2 environments. Nowadays, data from various sources (e.g., temperature and velocity), for both inhibited and non-inhibited CO2 solutions, can be fed into neural networks, allowing them to be used for data processing. An artificial neural network is proposed for the prediction of corrosion in the HAZ.A phenomenal outcome for the prediction of corrosion in the HAZ was proposed with the learning ability of an artificial neural network using software, through which training of 406 sets of data using the Leven Berg-Marquardt algorithm were obtained from experimental data. The training sets were developed for three levels of corrosion (mild, moderate and severe) through the Artificial Neural Network (ANN) and resulted in a trend which took the form of an incremental parabolic curve. This study presents an artificial neural network model which simulates the complex and nonlinear atmospheric corrosion process observed in experimental data. The correlation statistics (R) in the ANN proved to be 90% accurate. The test results were validated to confirm the efficacy of the developed ANN model for prediction of corrosion rate and good performance was observed. The interactions between the inputs were estimated by performing a sensitivity analysis based on the developed model. Since the model results from this research showed good agreement with experimentally obtained corrosion rates, it could now be widely applied in corrosion studies.
Keywords:
Artificial Neural Networks (ANN), corrosion , prediction,
References
-
Alawadhi, K. and M. Robinson, 2011. Preferential weld corrosion of X65 pipeline steel in flowing brines containing carbon dioxide. Corros. Eng. Sci. Techn., 46(4): 318-329.
-
ASTM G5-94, 2004. Standard Reference Test Method for Making Potentiostatic and Potentiodynamic Anodic Polarization Measurements.
Direct Link
-
ASTM G59-97, 2003. Standard Test Method for Conducting Potentiodynamic Polarization Resistance Measurements.
Direct Link
-
Chaves, I.A. and R.E. Melchers, 2014. External corrosion of carbon steel pipeline weld zones. Int. J. Offshore Polar, 24(1): 68-74.
-
De Masi, G., R. Vichi, M. Gentile, R. Bruschi and G. Gabetta, 2014. A neural network predictive model of pipeline internal corrosion profile. Proceeding of the 1st International Conference on Systems Informatics, Modelling and Simulation (SIMS '14), pp: 20-25.
-
Malinov, S., W. Sha and Z. Guo, 2000. Artificial neural network modelling of crystallization temperatures of the Ni–P based amorphous alloys material. Mater. Sci. Eng. A, 283: 1-218.
CrossRef
-
Metzbower, E.A., J.J. DeLoach, S.H. Lalam and H.K.D.H. Bhadeshia, 2001. Neural network analysis of strength and ductility of welding alloys for high strength low alloy shipbuilding steels. Sci. Technol. Weld. Joi., 6(2): 116-124.
-
Nafday, O.A. and S. Nesic, 2005. Iron carbonate scale formation and CO2corrosion in the presence of acetic acid. NACE Corrosion 2005, Paper No. 05295, NACE International.
-
Nyborg, R., 2005. Controlling internal corrosion in oil and gas pipelines. Bus. Brief. Explorat. Prod. Oil Gas Rev., 2: 70-74.
-
Oluwole, O. and N. Idusuyi, 2012. Artificial neural network modeling for al-zn-sn sacrificial anode protection of low carbon steel in saline media. Am. J. Mater. Sci., 2(3): 62-65.
-
Pardo, A., S. Feliu Jr., M.C. Merino, R. Arrabal and E. Matykina, 2009. Electrochemical estimation of the corrosion rate of magnesium/aluminium alloys. Int. J. Corrosion, 2010(2010): 8.
CrossRef Direct Link
-
Paul, S., 2012. Modeling to study the effect of environmental parameters on corrosion of mild steel in seawater using neural network. ISRN Metall., 2012(2012): 6.
-
Raja Dhas, J.E. and K. Somasundaram, 2013. Modeling and prediction of HAZ using finite element and neural network modeling. Adv. Prod. Eng. Manage., 8(1): 13-24.
-
Scully, J.R., 2000. Polarization resistance method for determination of instantaneous corrosion rates. Corrosion, 56(2): 199-218.
CrossRef Direct Link
-
Stansbury, E.E. and R.A. Buchanan, 2000. Fundamentals of Electrochemical Corrosion. ASM International, Materials Park, OH.
Direct Link
-
Turgoose, S. and J.W. Palmer, 2005. Preferential weld corrosion of 1% Ni welds: Effects of solution conductivity and corrosion inhibitors. Proceeding of CORROSION, 2005. NACE International, Houston, TX, USA.
Direct Link
Competing interests
The authors have no competing interests.
Open Access Policy
This article is distributed under the terms of the Creative Commons Attribution 4.0 International License (http://creativecommons.org/licenses/by/4.0/), which permits unrestricted use, distribution, and reproduction in any medium, provided you give appropriate credit to the original author(s) and the source, provide a link to the Creative Commons license, and indicate if changes were made.
Copyright
The authors have no competing interests.
|
|
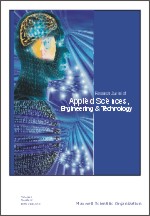 |
ISSN (Online): 2040-7467
ISSN (Print): 2040-7459 |
 |
Information |
|
|
|
Sales & Services |
|
|
|