Research Article | OPEN ACCESS
Identification and Inference of Cracks in Old Paintings Using Supervised Method
1S.P. Karuppiah and 2S.K. Srivatsa
1Jawaharlal Nehru Technological University, Hyderabad
2Prathyusha Institute of Technology and Management, Aranvoyalkuppam, Poonamallee-Tiruvallur Road, Tiruvallur-602 025, India
Research Journal of Applied Sciences, Engineering and Technology 2016 12:1176-1181
Received: March 12, 2015 | Accepted: May 22, 2015 | Published: June 15, 2016
Abstract
The older paintings are taken as input to find the crack and remove the crack using three steps: (a) identify crack (b) classify the crack (c) use trimmed median filter to get the quality of a rectified image. On many occasions the restoration of cracks in old paintings becomes a difficult task if it is done manually. So old paintings are digitized. It is evident that there is an increased need for carefully detailing the complexity of valuable sites with an improved accuracy. In the present paper a new effective methodology for digitizing the cracks that are caused by surrounding environment, particularly extreme changes in humidity and heat is presented. The digital paintings can be restored using different image processing techniques. When a painting is restored, the restorer must know which areas to be filled or recovered. MATLAB is used to build the code required to process and analyze the data. One of the most important findings of the paper is that the trimmed median filter technique is used to for the restoration of the digitized painting.
Keywords:
Cracks classification, image, painting, trimmed median filter,
References
-
Ballester, C., M. Bertalmio, V. Caselles, G. Sapiro and J. Verdera, 2001. Filling-in by joint interpolation of vector fields and gray levels. IEEE T. Image Process., 10(8): 1200-1211.
CrossRef
-
Barni, M., F. Bartolini and V. Cappellini, 2000. Image processing for virtual restoration of artworks. IEEE Multimedia, 7(2): 34-37.
CrossRef
-
Bors, A.G. and I. Pitas, 1996. Median radial basis function neural network. IEEE T. Neural Networ., 7(6): 1351-1364.
CrossRef PMid:18263530
-
Chan, T.F. and J. Shen, 2001. Nontexture inpainting by curvature-driven diffusions. J. Vis. Commun. Image R., 12(4): 436-449.
CrossRef
-
Elberly, D., R. Gardner, B. Morse, S. Pizer and C. Scharlach, 1994. Ridges for image analysis. J. Math. Imaging Vis., 4(4): 353-373.
CrossRef
-
Esedoglu, S. and J. Shen, 2002. Digital inpainting based on the Mumford-Shah-Euler image model. Eur. J. Appl. Math., 13: 353-370.
CrossRef
-
Gauch, J.M. and S.M. Pizer, 1993. Multiresolution analysis of ridges and valleys in grey-scale images. IEEE T. Pattern Anal., 15(6): 635-646.
CrossRef
-
Giakoumis, I., N. Nikolaidis and I. Pitas, 2006. Digital image processing techniques for the detection and removal of cracks in digitized paintings. IEEE T. Image Process., 15(1): 178-188.
CrossRef PMid:16435548
-
Gupta, A., V. Khandelwal, A. Gupta and M.C. Srivastava, 2008. Image processing methods for the restoration of digitized paintings. Thammasat Int. J. Sci. Technol., 13(3): 66-72.
-
Haralick, R.M., 1983. Ridges and valleys on digital images. Comput. Vision Graph. Image Process., 22(1): 28-38.
CrossRef
-
Karuppiah, S.P. and S.K. Srivatsa, 2012. Recognition and inference of crevice processing on digitized paintings. Proceeding of the SPIE 8768, International Conference on Graphic and Image Processing, pp: 1-7.
-
Karuppiah, S.P. and S.K. Srivatsa, 2014. An innovative morphological approach for noise removal cum edge detection for digitized paintings. J. Comput. Sci., 10(8): 1569-1574.
CrossRef
-
Kokaram, A.C., R.D. Morris, W.J. Fitzgerald and P.J.W. Rayner, 1995. Interpolation of missing data in image sequences. IEEE T. Image Process., 4(11): 1509-1519.
CrossRef PMid:18291983
-
Lopez, A.M., F. Lumbreras, J. Serrat and J.J. Villanueva, 1999. Evaluation of methods for ridge and valley detection. IEEE T. Pattern Anal., 21(4): 327-335.
CrossRef
-
Pitas, I., C. Kotropoulos, N. Nikolaidis, R. Yang and M. Gabbouj, 1996. Order statistics learning vector quantizer. IEEE T. Image Process., 5(6): 1048-1053.
CrossRef PMid:18285191
-
Rajashekharan, S. and G.A. Vijyalaxmi Pai, 2003. Neural Networks, Fuzzy Logic, and Genetic Algorithms: Synthesis and Applications. 6th Edn., Prentice-Hall of India, New Dehli, pp: 34-86.
-
Solanki, S.V. and A.R. Mahajan, 2009. Cracks inspection and interpolation in digitized artistic picture using image processing approach. Int. J. Recent Trends Eng., 1(2): 97-99.
-
Yao, J., M. Dash, S.T. Tan and H. Liu, 2000. Entropy-based fuzzy clustering and fuzzy modeling. Fuzzy Set. Syst., 113(3): 381-388.
CrossRef
Competing interests
The authors have no competing interests.
Open Access Policy
This article is distributed under the terms of the Creative Commons Attribution 4.0 International License (http://creativecommons.org/licenses/by/4.0/), which permits unrestricted use, distribution, and reproduction in any medium, provided you give appropriate credit to the original author(s) and the source, provide a link to the Creative Commons license, and indicate if changes were made.
Copyright
The authors have no competing interests.
|
|
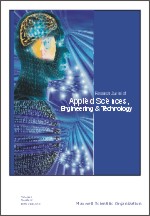 |
ISSN (Online): 2040-7467
ISSN (Print): 2040-7459 |
 |
Information |
|
|
|
Sales & Services |
|
|
|