Research Article | OPEN ACCESS
A Committee Machine with Intelligent Systems for Estimating Monthly Mean Reference Evapotranspiration in an Arid Region
1Ali H. Al-Aboodi, 1Alaa M. Al-Abadi and 1Husham T. Ibrahim
1Department of Civil Engineering, College of Engineering, University of Basrah, Basrah, Iraq
2Department of Geology, College of Sciences, University of Basrah, Basrah, Iraq
Research Journal of Applied Sciences, Engineering and Technology 2017 10:386-398
Received: May 15, 2017 | Accepted: June 23, 2017 | Published: October 15, 2017
Abstract
The aim of this research is to estimate the reference evapotranspiration ETo as given by FAO-56 PM equation in Basrah city, southern Iraq by using several climatic inputs data including maximum monthly mean air temperature, minimum monthly mean air temperature, monthly mean relative humidity and monthly mean wind speed. Three artificial intelligent systems (generalized regression neural network GRNN, multi-layer perceptron MLP and adaptive neuro-fuzzy inference systems ANFIS) were used for predicting reference evapotranspiration. Root mean squared error and coefficient of determination were used as comparison criteria for evaluation of performance of all the developed models. The results shown that the models performances of multi-layer perceptron models are better than adaptive neuro-fuzzy inference systems models and slightly better than generalized regression neural network models with different inputs combination. A Committee Machine with Intelligent Systems (CMIS) was constructed for estimation of ETo by integrating the results of predicting ETo from GRNN, MLP and ANFIS, each of them has a weight factor representing its contribution in overall estimation. The results illustrated that the performance of committee machine with intelligent systems is better than any one of the individual artificial intelligent systems for predicting ETo.
Keywords:
Adaptive neuro-fuzzy inference systems, artificial neural network, basrah, committee machine, evapotranspiration, Iraq,
References
- Allen, R.G., L.S. Pereira, D. Raes and M. Smith, 1998. Crop evapotranspiration: Guidelines for computing crop water requirements. Irrigation and Drainage Paper No. 56, FAO, Rome, Italy.
- Specht, D.F., 1991. A general regression neural network. IEEE T. Neural Networ., 2(6): 568-576.
CrossRef PMid:18282872 -
Alilou, V.K. and F. Yaghmaee, 2015. Application of GRNN neural network in non-texture image inpainting and restoration. Pattern Recogn. Lett., 62: 24-31.
CrossRef - Bagheripour, P. and M. Asoodeh, 2014. Committee machine reaping of three well-known models: Established between saturation pressure and gas chromatography data. Appl. Petrochem. Res., 4(3): 313-316.
CrossRef - Barzegar, R., M. Sattarpour, M.R. Nikudel and A.A. Moghaddam, 2016. Comparative evaluation of artificial intelligence models for prediction of uniaxial compressive strength of travertine rocks, case study: Asarshahr area, NW Iran. Model Earth Syst. Environ., 2: 76.
CrossRef -
Bhatt, A. and H.B. Helle, 2002. Committee neural networks for porosity and permeability prediction from well logs. Geophys. Prospect., 50(6): 645-660.
CrossRef - Chen, C.H. and Z.S. Lin, 2006. A committee machine with empirical formulas for permeability prediction. Comput. Geosci., 32(4): 485-496.
CrossRef -
Disorntetiwat, P., 2001. Global stock index forecasting using multiple generalized regression neural networks with a gating network. Ph.D. Thesis, University of Missouri Rolla, Missouri, United States.
- Elleithy, K., 2010. Advanced Techniques in Computing Sciences and Software Engineering. Springer, Netherlands, pp: 599.
CrossRef -
Gavilan, P., I.J. Lorite, S. Tornero and J. Berengena, 2006. Regional calibration of Hargreaves equation for estimating reference ET in a semiarid environment. Agr. Water Manage., 81(3): 257-281.
CrossRef - Ghiasi-Freez, J., A. Kadkhodaie-Ilkhchi and M. Ziaii, 2012. Improving the accuracy of flow units prediction through two committee machine models: An example from the South Pars Gas Field, Persian Gulf Basin, Iran. Comput. Geosci., 46: 10-23.
CrossRef - Hannan, S.A., R.R. Manza and R.J. Ramteke, 2010. Generalized regression neural network and radial basis function for heart disease diagnosis. Int. J. Comput. Appl., 7(13): 7-13.
CrossRef - Haykin, S., 1991. Neural Networks: A Comprehensive Foundation. Prentice-Hall, Englewood Cliffs, pp: 842.
- Hossen, J., S. Sayeed, I. Yusof and S.M.A. Kalaiarasi, 2013. A framework of modified adaptive fuzzy inference engine (MAFIE) and its application. Int. J. Comput. Inform. Syst. Ind. Manage. Appl., 5: 662-670.
Direct Link -
Kadkhodaie-Ilkhchi, A., M.R. Rezaee and H. Rahimpour-Bonab, 2009. A committee neural network for prediction of normalized oil content from well log data: An example from South Pars Gas Field, Persian Gulf. J. Petrol. Sci. Eng., 65(1-2): 23-32.
CrossRef - Karunanidhi, N., W.J. Grenney, D. Whitley and K. Bovee, 1994. Neural networks for river flow prediction. J. Comput. Civil Eng., 8(2): 201-219.
CrossRef - Kisi, O., 2007. Stream?ow forecasting using different arti?cial neural network algorithms. J. Hydrol. Eng., 12(5): 532-539.
CrossRef - Kisi, O., M. Tombul and M.Z. Kermani, 2015. Modeling soil temperatures at different depths by using three different neural computing techniques. Theor. Appl. Climatol., 121(1-2): 377-387.
CrossRef -
Kumar, M., N.S. Raghuwanshi, R. Singh, W.W. Wallender and W.O. Pruitt 2002. Estimating evapotranspiration using artificial neural network. J. Irrig. Drain. Eng., 128(4): 224-233.
CrossRef - Kumar, R., V. Shankar and M. Kumar, 2011. Modeling of crop reference evapotranspiration: A review. Univer. J. Environ. Res. Technol., 1(3): 239-246.
Direct Link - Landeras, G., A. Ortiz-Barredo and J.J. L�pez, 2008. Comparison of artificial neural network models and empirical and semi-empirical equations for daily reference evapotranspiration estimation in the Basque Country (Northern Spain). Agr. Water Manage., 95(5): 553-565.
CrossRef - Lim, J.S., 2005. Reservoir properties determination using fuzzy logic and neural networks from well data in offshore Korea. J. Petrol. Sci. Eng., 49(3-4): 182-192.
CrossRef -
L�pez-Urrea, R., F. Martin de Santa Olalla, C. Fabeiro and A. Moratalla, 2006. Testing evapotranspiration equations using lysimeter observations in a semiarid climate. Agr. Water Manage., 85(1-2): 15-26.
CrossRef - Loukas, Y.L., 2001. Adaptive neuro-fuzzy inference system: An instant and architecture-free predictor for improved QSAR studies. J. Med. Chem., 44(17): 2772-2783.
CrossRef PMid:11495588 - Nourani, V. and A. Babakhani, 2013. Integration of artificial neural networks with radial basis function interpolation in earthfill dam seepage modeling. J. Comput. Civil Eng., 27(2): 183-195.
CrossRef - Pereira, A.R. and W.O. Pruitt, 2004. Adaptation of the Thornthwaite scheme for estimating daily reference evapotranspiration. Agr. Water Manage., 66(3): 251-257.
CrossRef - Rumelhart, D.E. and J.L. McClelland, 1986. Parallel Distributed Processing: Explorations in the Microstructure of Cognition. MIT Press, Cambridge, Mass.
Direct Link - Stoffel, K., P. Cotofrei and D. Han, 2012. Fuzzy clustering based methodology for multidimensional data analysis in computational forensic domain. Int. J. Comput. Inform. Syst. Ind. Manage. Appl., 4: 400-410.
Direct Link - Tang, X.G., H.P. Li, A.R. Desai, Z. Nagy, J.H. Luo, T.E. Kolb, A. Olioso, X.B. Xu, L. Yao, W. Kutsch, K. Pilegaard, B. K�stner and C. Ammann, 2014. How is water-use efficiency of terrestrial ecosystems distributed and changing on Earth? Sci. Rep-UK, Vol. 4, Article No. 7483.
Direct Link - Trajkovic, S. and S. Kolakovic, 2009. Evaluation of reference evapotranspiration equations under humid conditions. Water Resour. Manag., 23: 3057-3067.
CrossRef - Yu, W.C., 1979. Positive basis and a class of direct search techniques. Sci. Sin. Math., 9(1): 53-67.
Competing interests
The authors have no competing interests.
Open Access Policy
This article is distributed under the terms of the Creative Commons Attribution 4.0 International License (http://creativecommons.org/licenses/by/4.0/), which permits unrestricted use, distribution, and reproduction in any medium, provided you give appropriate credit to the original author(s) and the source, provide a link to the Creative Commons license, and indicate if changes were made.
Copyright
The authors have no competing interests.
|
|
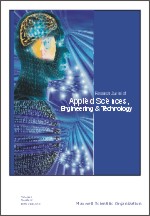 |
ISSN (Online): 2040-7467
ISSN (Print): 2040-7459 |
 |
Information |
|
|
|
Sales & Services |
|
|
|