Research Article | OPEN ACCESS
A Novel GMM-Based Feature Reduction for Vocal Fold Pathology Diagnosis
Vahid Majidnezhad and Igor Kheidorov
Department of Computer Engineering, Shabestar Branch, Islamic Azad University, Shabestar, Iran
Research Journal of Applied Sciences, Engineering and Technology 2013 6:2245-2254
Received: September 11, 2012 | Accepted: October 19, 2012 | Published: February 21, 2013
Abstract
Acoustic analysis is a proper method in vocal fold pathology diagnosis so that it can complement and in some cases replace the other invasive, based on direct vocal fold observation, methods. There are different approaches and algorithms for vocal fold pathology diagnosis. These algorithms usually have three stages which are Feature Extraction, Feature Reduction and Classification. While the third stage implies a choice of a variety of machine learning methods (Support Vector Machines, Gaussian Mixture Model, etc.), the first and second stages play a critical role in performance and accuracy of the classification system. In this study we present initial study of feature extraction and feature reduction in the task of vocal fold pathology diagnosis. A new type of feature vector, based on wavelet packet decomposition and Mel-Frequency-Cepstral-Coefficients (MFCCs), is proposed. Also a new method for feature reduction is proposed and compared with conventional methods such as Principal Component Analysis (PCA), F-Ratio and Fisher’s discriminant ratio. Gaussian Mixture Model is used as a classifier for evaluating the performance of the proposed method. The results show the priority of the proposed method in comparison with current methods.
Keywords:
Gaussian Mixture Model (GMM), Mel-Frequency-Cepstral-Coefficient (MFCC), Principal Component Analysis (PCA), proposed GMM-based feature reduction, vocal fold pathology diagnosis, wavelet packet decomposition,
Competing interests
The authors have no competing interests.
Open Access Policy
This article is distributed under the terms of the Creative Commons Attribution 4.0 International License (http://creativecommons.org/licenses/by/4.0/), which permits unrestricted use, distribution, and reproduction in any medium, provided you give appropriate credit to the original author(s) and the source, provide a link to the Creative Commons license, and indicate if changes were made.
Copyright
The authors have no competing interests.
|
|
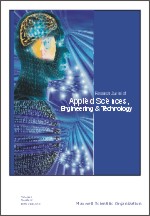 |
ISSN (Online): 2040-7467
ISSN (Print): 2040-7459 |
 |
Information |
|
|
|
Sales & Services |
|
|
|