Research Article | OPEN ACCESS
Learning from Adaptive Neural Control of Electrically-Driven Mechanical Systems
Yu-Xiang Wu, Jing Zhang and Cong Wang
College of Automation Science and Engineering, South China University of Technology, Guangzhou 510640, China
Research Journal of Applied Sciences, Engineering and Technology 2013 16:3034-3043
Received: January 10, 2013 | Accepted: January 31, 2013 | Published: September 10, 2013
Abstract
This study presents deterministic learning from adaptive neural control of unknown electrically-driven mechanical systems. An adaptive neural network system and a high-gain observer are employed to derive the controller. The stable adaptive tuning laws of network weights are derived in the sense of the Lyapunov stability theory. It is rigorously shown that the convergence of partial network weights to their optimal values and locally accurate NN approximation of the unknown closed-loop system dynamics can be achieved in a stable control process because partial Persistent Excitation (PE) condition of some internal signals in the closed-loop system is satisfied. The learned knowledge stored as a set of constant neural weights can be used to improve the control performance and can also be reused in the same or similar control task. Numerical simulation is presented to show the effectiveness of the proposed control scheme.
Keywords:
Adaptive neural control, deterministic learning, electrically-driven mechanical systems, high-gain observer, RBF network,
Competing interests
The authors have no competing interests.
Open Access Policy
This article is distributed under the terms of the Creative Commons Attribution 4.0 International License (http://creativecommons.org/licenses/by/4.0/), which permits unrestricted use, distribution, and reproduction in any medium, provided you give appropriate credit to the original author(s) and the source, provide a link to the Creative Commons license, and indicate if changes were made.
Copyright
The authors have no competing interests.
|
|
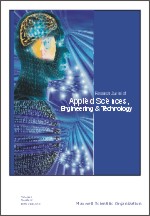 |
ISSN (Online): 2040-7467
ISSN (Print): 2040-7459 |
 |
Information |
|
|
|
Sales & Services |
|
|
|