Research Article | OPEN ACCESS
A Novel Fault Diagnosis Method for Rolling Element Bearings Using Kernel Independent Component Analysis and Genetic Algorithm Optimized RBF Network
Hang Dai and Jingshi He
Dongguan Polytechnic, Dongguan 523808, China
Research Journal of Applied Sciences, Engineering and Technology 2013 5:895-899
Received: October 30, 2012 | Accepted: December 15, 2012 | Published: June 25, 2013
Abstract
This study focuses on the defect detection of rolling element bearings using a novel method. In the bearing fault diagnosis, the fault feature extraction is also a key issue for successful fault detection. However, the vibration signals acquired by accelerometers are often mixed with noise signals. These mixtures may influence the fault feature extraction and hence deteriorate the performance of the fault diagnosis. To address this problem, a novel method is presented in this study to enhance the performance of the fault diagnosis of rolling element bearings. In this new method, the Kernel Independent Component Analysis (KICA) was firstly employed to fuse multi-sensor signals to eliminate noise signals. Then a RBF network was used to classify the fault patterns. To improve the fault identification, the Genetic Algorithm (GA) was adopted to optimize the parameters of the RBF network. Experiment tests on a rolling bearing fault diagnosis set-up have been carried out to verify the performance of the proposed method. The test results show that show the KICA can remove the noise effectively and the GA optimized RBF network can provide accurate fault detection results. In addition, the new method has been compared with the single RBF network model and ICA-RBF model. The comparison indicates that the proposed KICA-RBF model outperforms the other two rivals with a fault detection rate of 92.3%.
Keywords:
Fault diagnosis, KICA, RBF, rolling element bearings,
Competing interests
The authors have no competing interests.
Open Access Policy
This article is distributed under the terms of the Creative Commons Attribution 4.0 International License (http://creativecommons.org/licenses/by/4.0/), which permits unrestricted use, distribution, and reproduction in any medium, provided you give appropriate credit to the original author(s) and the source, provide a link to the Creative Commons license, and indicate if changes were made.
Copyright
The authors have no competing interests.
|
|
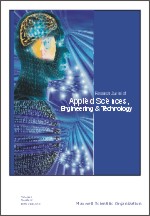 |
ISSN (Online): 2040-7467
ISSN (Print): 2040-7459 |
 |
Information |
|
|
|
Sales & Services |
|
|
|