Research Article | OPEN ACCESS
Model Reference Adaptive Control Based on GANN for Vertical Electric Furnace
1Hongxing Li, 2Xiangling Kong and 1Yinong Zhang
1Automation College
2Information College, Beijing Union University, Beijing 100101, P.R. China
Research Journal of Applied Sciences, Engineering and Technology 2014 8:1529-1535
Received: May 10, 2013 | Accepted: June 07, 2013 | Published: February 27, 2014
Abstract
The vertical electric furnace is a multi-variable complex system, conventional control methods are used to control it, to need modelling and decoupling. In this study, a model reference adaptive control using the Neural Network with Genetic Algorithm (GANN) for the temperature control of the vertical electric furnace is proposed. The neural model of the system is identified by the genetic algorithm. Another neural network is trained to learn the inverse dynamics of the system so that it can be used as a nonlinear controller. Because of the limitation of BP algorithm, the genetic algorithm is used to find the fitness weights and thresholds of the neural network model and the simulation results show that the model is satisfied and the control is effective.
Keywords:
Adaptive control, genetic algorithm, multi-variable system, neural network, vertical electric furnace,
References
-
Araz, O.U. and L. Salum, 2010. A multi-criteria adaptive control scheme based on neural networks and fuzzy inference for DRC manufacturing systems. Int. J. Prod. Res., 48(1): 251-270.
CrossRef
-
Goldberg, D.E., 1989. Genetic Algorithms in Search, Optimization and Machine Learning. Addison-Wesley, Reading, Massachusetts.
-
Hornik, K., M. Stincheombe and H. White, 1989. Multilayer feed forward networks are universal approximators. Neural Networks, 2: 359-366.
CrossRef
-
Hsu, C.F., C.M. Lin and T.Y. Chen, 2005. Neural network identification based adaptive control of wing rock motions. IEE P-Contr. Theor. Ap., 152(1): 65-71.
-
Li, H.X., J.S. Lu and H.S. Yan, 2005. Model reduction using the genetic algorithm and Routh approximations. J. Syst. Eng. Electr., 16(3): 632-639.
-
Sharma, S.K., S.F. McLoone and G.W. Irwin, 2005. Genetic algorithms for local controller network construction. IEE P-Contr. Theor. Ap., 152(5): 587-597.
-
Teng, T.K., J.S. Shieh and C.S. Chen, 2003. Genetic algorithm applied in online autotuning PID parameters of a liquid-level control system. T. I. Meas. Control, 25(5): 433-450.
CrossRef
-
Wang, X.S., H. Hong and C.Y. Su, 2003. Model reference adaptive control of continuous-time systems with an unknown input dead-zone. IEE P-Contr. Theor. Ap., 150(3): 261-266.
Competing interests
The authors have no competing interests.
Open Access Policy
This article is distributed under the terms of the Creative Commons Attribution 4.0 International License (http://creativecommons.org/licenses/by/4.0/), which permits unrestricted use, distribution, and reproduction in any medium, provided you give appropriate credit to the original author(s) and the source, provide a link to the Creative Commons license, and indicate if changes were made.
Copyright
The authors have no competing interests.
|
|
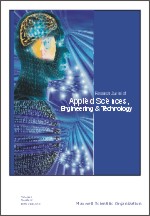 |
ISSN (Online): 2040-7467
ISSN (Print): 2040-7459 |
 |
Information |
|
|
|
Sales & Services |
|
|
|