Research Article | OPEN ACCESS
Fuzzy and Regression Modelling of Hard Milling Process
A. Tamilarasan and K. Marimuthu
Department of Mechanical Engineering, Coimbatore Institute of Technology,
Civil Aerodrome Post, Coimbatore, 641014, Tamilnadu, India
Research Journal of Applied Sciences, Engineering and Technology 2014 16:3380-3386
Received: October 19, 2013 | Accepted: October 28, 2013 | Published: April 25, 2014
Abstract
The present study highlights the application of box-behnken design coupled with fuzzy and regression modeling approach for making expert system in hard milling process to improve the process performance with systematic reduction of production cost. The important input fields of work piece hardness, nose radius, feed per tooth, radial depth of cut and axial depth cut were considered. The cutting forces, work surface temperature and sound pressure level were identified as key index of machining outputs. The results indicate that the fuzzy logic and regression modeling technique can be effectively used for the prediction of desired responses with less average error variation. Predicted results were verified by experiments and shown the good potential characteristics of the developed system for automated machining environment.
Keywords:
Box-behnken design, cutting forces, fuzzy logic, hard milling, sound, temperature,
References
-
Ali, Y.M. and L.C. Zhang, 2004. A fuzzy model for predicting burns in surface grinding of steel. Int. J. Mach. Tool. Manu., 44: 563-571.
CrossRef -
Cabrera, F.M., E. Beamud, I. Hanafi, A. Khamlichi and A. Jabbouri, 2011. Fuzzy Logic-based modeling of surface roughness parameters for CNC turning of PEEK CF30 by TiN-coated cutting tools. J. Thermoplast. Compos., 24: 399-413.
CrossRef -
Cahskan, H., C. Kurbanoglu, P. Panjan and D. Kramar, 2013. Investigation of the performance of carbide cutting tools with hard coatings in hard milling based on the response surface methodology. Int. J. Adv. Manuf. Tech., 66: 883-893.
CrossRef -
Chakravarthy, S.P. and R.N. Babu, 2000. A hybrid approach for selection of optimal process parameters in abrasive water jet cutting. J. Eng. Manuf., 214: 781-791.
CrossRef -
Chun, K.C., A.J. Shih and S.F. Miller, 2008. Fuzzy logic control of microhole electrical discharge machining. J. Manuf. Sci. Eng., 130: 064502-06.
CrossRef -
Ding, T., S. Zhang, Y. Wang and X. Zhu, 2010. Empirical models and optimal cutting parameters for cutting forces and surface roughness in hard milling of AISI H13 steel. Int. J. Adv. Manuf. Tech., 51: 45-55.
CrossRef -
Dureja, J.S., V.K. Gupta, V.S. Sharma and M. Dogra, 2009. Design optimization of cutting conditions and analysis of their effect on tool wear and surface roughness during hard turning of AISI-H11 steel with a coated-mixed ceramic tool. J. Eng. Manuf., 223: 1441-1453.
CrossRef -
Gopalsamy, B.M., B. Mondal, S. Ghosh, K. Arntz and F. Klocke, 2010. Experimental investigations while hard machining of DIEVAR tool steel (50 HRC). Int. J. Adv. Manuf. Tech., 51: 853-869.
CrossRef -
Hanafi, I., A. Khamlichi, F.M. Cabrera, P.J. Nu-ezLópez and A. Jabbouri, 2012. Fuzzy rule based predictive model for cutting force in turning of reinforced PEEK composite. Measurement, 45: 1424-1435.
CrossRef -
Iqbal, A., N. He, N.U. Dar and L. Li, 2007a. Comparison of fuzzy expert system based strategies of off line and online estimation of flank wear in hard milling process. Expert Syst. Appl., 33: 61-66.
CrossRef -
Iqbal, A., N. He, N.U. Dar and L. Li, 2007b. A fuzzy expert system for optimizing parameters and predicting performance measures in hard-milling process. Expert Syst. Appl., 32: 1020-1027.
CrossRef -
Kovac, P., D. Rodic, V. Pucovsky, B. Savkovic and M. Gostimirovic, 2013. Application of fuzzy logic and regression analysis for modeling surface roughness in face milling. J. Intell. Manuf., 24: 755-762.
CrossRef -
Labib, A.W., V.J. Keasberry, J. Atkinson and H.W. Frost, 2011. Towards next generation electrochemical machining controllers: A fuzzy logic control approach to ECM. Expert Syst. Appl., 38: 7486-7493.
CrossRef -
Latha, B. and V.S. Senthilkumar, 2009. Fuzzy rule based modeling of drilling parameters for delamination in drilling of GFRP composites. J. Reinf. Plast. Comp., 28(8): 951-964.
CrossRef -
Montgomery, D.C., 2012. Design and Analysis of Experiments. 8th Edn., Wiley, New York.
-
Özek, C., U. Çaydas and E. Ünal, 2012. A fuzzy model for predicting surface roughness in plasma arc cutting of AISI 4140 steel. Mater. Manuf. Process., 27(1): 95-102.
CrossRef -
Ozel, T., T.K. Hsu and E. Zeren, 2005. Effects of cutting edge geometry, work piece hardness, feed rate and cutting speed on surface roughness and forces in finish turning of hardened AISI H13 steel. Int. J. Adv. Manuf. Tech., 25: 262-269.
CrossRef -
Pandey, A.K and A.K. Dubey, 2012. Taguchi based fuzzy logic optimization of multiple quality characteristics in laser cutting of Duralumin sheet. Opt. Laser. Eng., 50: 328-335.
CrossRef -
Rajasekaran, T., K. Palanikumar and B.K. Vinayagam, 2011. Application of fuzzy logic for modeling surface roughness in turning CFRP composites using CBN tool. Prod. Eng. Res. Devel., 5: 191-199.
CrossRef -
Ramesh, S., L. Karunamoorthy and K. Palanikumar, 2008. Fuzzy modeling and analysis of machining parameters in machining titanium alloy. Mater. Manuf. Process., 23(4): 439-447.
CrossRef -
Singh, A., S. Datta, S.S. Mahapatra, T. Singha and G. Majumdar, 2011. Optimization of bead geometry of submerged arc weld using fuzzy based desirability function approach. J. Intell. Manuf., 24: 35-44.
CrossRef -
Vundavilli, P.R., M.B. Parappagoudar, S.P. Kodali and S. Benguluri, 2012. Fuzzy logic-based expert system for prediction of depth of cut in abrasive water jet machining process. Knowl-Based Syst., 27: 456-464.
CrossRef -
Zadeh, L.A., 1965. Fuzzy sets. Inform. Control, 8: 338-353.
CrossRef -
Zhai, L.F., M. Sun, W. Song and G. Wang, 2012. An integrated approach to optimize the conditioning chemicals for enhanced sludge conditioning in a pilot-scale sludge dewatering process. Bioresour. Technol., 121: 161-168.
CrossRef PMid:22858481
Competing interests
The authors have no competing interests.
Open Access Policy
This article is distributed under the terms of the Creative Commons Attribution 4.0 International License (http://creativecommons.org/licenses/by/4.0/), which permits unrestricted use, distribution, and reproduction in any medium, provided you give appropriate credit to the original author(s) and the source, provide a link to the Creative Commons license, and indicate if changes were made.
Copyright
The authors have no competing interests.
|
|
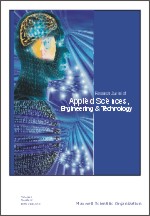 |
ISSN (Online): 2040-7467
ISSN (Print): 2040-7459 |
 |
Information |
|
|
|
Sales & Services |
|
|
|