Research Article | OPEN ACCESS
Optimization of Ferrite Number of Solution Annealed Duplex Stainless Steel Cladding Using Integrated Artificial Neural Network: Simulated Annealing
1V. Rathinam and 2T. Kannan
1Department of Automobile Engineering, Paavaai Group of Institutions,
Namakkal 637018, Tamilnadu, India
2S.V.S. College of Engineering, Coimbatore 642109, Tamilnadu, India
Research Journal of Applied Sciences, Engineering and Technology 2014 21:4464-4475
Received: December 09, 2013 | Accepted: December 20, 2013 | Published: June 05, 2014
Abstract
Cladding is the most economical process used on the surface of low carbon structural steel to improve the corrosion resistance. The corrosion resistant property is based on the amount of ferrite present in the clad layer. Generally, the ferrite content present in the layer is expressed in terms of Ferrite Number (FN). The optimum range of ferrite number provides adequate surface properties like chloride stress corrosion cracking resistance, pitting and crevice corrosion resistance and mechanical properties. For achieving maximum economy and enhanced life, duplex stainless steel (E2209T1-4/1) is deposited on the surface of low carbon structural steel of IS: 2062. The problem faced in the weld cladding towards achieving the required amount of ferrite number is selection of optimum combination of input process parameters. This study concentrates on estimating FN and analysis of input process parameters on FN of heat treated duplex stainless steel cladding. To predict FN, mathematical equations were developed based on four factor five level central composite rotatable design with full replication using regression methods. Then, the developed models were embedded further into integrated ANN-SA to estimate FN. From the results, the integrated ANN-SA is capable of giving maximum FN at optimum process parameters compared to that of experimental, regression and ANN modeling.
Keywords:
Artificial neural network, duplex stainless steel, ferrite number, flux cored arc welding, simulated annealing, solution annealing heat treatment,
References
-
Azlan, M.Z., H. Habibollah and S. Safian, 2011. Estimation of the minimum Machining performance in the abrasive water jet machining using integrated ANN-SA. Expert Syst. Appl., 38(7): 8316-8326.
CrossRef
-
Badji, R., B. Belkessa, H. Maza, M. Bouabdallah, B. Bacroix and C. Kahloun, 2004. Effect of post weld heat treatment on microstructure and mechanical properties of welded 2205 duplex stainless steel. Mater. Sci. Forum., 467: 217-222.
CrossRef
-
Badji, R. M. Bouabdallah, B. Bacroix, C. Kahloun, B. Belkessa and H. Maza, 2008. Phase transformation and mechanical behavior in annealed 2205 duplex stainless steel welds. Mater. Charact., 59(4): 447-453.
CrossRef
-
Cochran, W.G. and G.M. Cox, 1987. Experimental Designs. John Wiley and Sons, NY, pp: 370.
-
Heejoon, H. and P. Yongsoo, 2009. Effects of heat treatment on the phase ratio and corrosion resistance of duplex stainless steel. Mater. Trans., 50: 1548-1552.
CrossRef
-
Kannan, T. and Dr. N. Murugan, 2005. Optimization of FCAW process parameters in duplex stainless steel weld cladding. Manuf. Technol. Today, 4(4): 3-7.
-
Karlsson, L., 1999. Intermetallic phase precipitation in duplex stainless steels and weld metals: Metallurgy, influence on properties and welding aspects. Weld. World, 43(5): 20-41.
-
Karlsson, L., L. Ryen and S. Pak, 1995. Precipitation of intermetallic phase in 22% Cr duplex stainless steel weld metals. Weld. J., 74: 28s-40s.
-
Kim, D., S. Rhee and H. Park, 2002. Modelling and optimization of a GMAW welding process by genetic algorithm and response surface methodology. Int. J. Prod. Res., 40(7): 1699-1711.
CrossRef
-
Kim, I.S., J.S. Son, C.E. Park, C.W. Lee and Y.K.D.V. Prasad, 2002. A study on Prediction of weld bead Height in robotic arc welding using a neural network. J. Mater. Process. Tech., 130: 229-234.
CrossRef
-
Kirkpatrick, F., C.D. Gelatt Jr. and M.P. Vecchi, 1983. Optimization by simulated annealing. Science, 220(4598): 671-780.
CrossRef PMid:17813860
-
Kotecki, D.J., 1989. Heat treatment of duplex stainless steel weld metals. Weld. J., 1989: 431s-440s.
-
Kotecki, D.J., 1997. Ferrite determination in stainless steel welds: Advances since 1974. Weld. J., 76(1): 24-s-36s.
-
Lai, J.K.L., K.W. Wong and D.J. Li, 1995. Effect of solution treatment on the transformation behavior of cold-rolled duplex stainless steels. Mater. Sci. Eng., 203: 356-364.
CrossRef
-
Montgomery, D.C., 2003. Design and Analysis of Experiments. 5th Edn., John Wiley and Sons, India, pp: 429.
-
Murugan, N. and R.S. Parmer, 1994. Effects of MIG process parameters on the geometry of the bead in the automatic surfacing of stainless steel. J. Mater. Process. Tech., 41: 381-398.
CrossRef
-
Murugan, N. and P.K. Palani, 2004. Optimization of bead geometry in automatic stainless steel cladding by MIG welding using a Genetic Algorithm. J. Inst. Eng., 84: 49-54.
-
Nagesh, D.S. and G.L. Datta, 2002. Prediction of weld bead geometry and penetration in shielded metal arc welding using artificial neural network. J. Mater. Process. Tech., 123: 303-312.
CrossRef
-
Nagesh, D.S. and G.L. Datta, 2010. Genetic algorithm for optimization of welding variables for height to width ratio and application of ANN for prediction of bead geometry for TIG welding process. Appl. Soft Comput., 10: 897-907.
CrossRef
-
Palani, P.K. and N. Murugan, 2007. Optimization of weld bead geometry for stainless steel cladding deposited by FCAW. J. Mater. Process. Tech., 190: 291-299.
CrossRef
-
Parikshit, D. and K.P. Dilip, 2007. Modeling of TIG welding process using conventional regression analysis and neural network-based approaches. J. Mater. Process. Tech., 184: 56-68.
CrossRef
-
Ping, L., M.T.C. Fang and J. Lucas, 1997. Modeling of submerged arc weld beads using self-adaptive offset neural networks. J. Mater. Process. Tech., 71: 288-298.
CrossRef
-
Vasudevan, M., A.K. Bhaduri, R. Baldev and K. Prasad Rao, 2003. Delta ferrite prediction in stainless steel welds using neural network analysis and comparison with other prediction methods. J. Mater. Process. Tech., 142: 20-28.
CrossRef
-
Vidyut, D., K.P. Dilip, G.L. Datta, M.N. Jha, T.K. Saha and A.V. Bapat, 2009. Optimization of bead geometry in electron beam welding using a genetic algorithm. J. Mater. Process. Tech., 209(3): 1151-1157.
CrossRef
-
Vitek, J.M., Y.S. Iskander and E.M. Oblow, 2000. Improved ferrite number prediction in stainless steel arc welds using artificial neural network: Part 2: Neural network results. Weld. Res. Suppl., 79(2): 41s-49.
-
Yoganandh, J., T. Kannan, S.P. Kumaresh Babu and S. Natarajan, 2012. Optimization of GMAW process parameters in Austenitic stainless steel cladding using Genetic algorithm based computational models. Exp. Techniques, 37(5): 48-58.
CrossRef
Competing interests
The authors have no competing interests.
Open Access Policy
This article is distributed under the terms of the Creative Commons Attribution 4.0 International License (http://creativecommons.org/licenses/by/4.0/), which permits unrestricted use, distribution, and reproduction in any medium, provided you give appropriate credit to the original author(s) and the source, provide a link to the Creative Commons license, and indicate if changes were made.
Copyright
The authors have no competing interests.
|
|
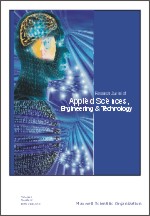 |
ISSN (Online): 2040-7467
ISSN (Print): 2040-7459 |
 |
Information |
|
|
|
Sales & Services |
|
|
|