Research Article | OPEN ACCESS
Classification of EMG Signal Based on Human Percentile using SOM
M.H. Jali, Z.H. Bohari, M.F. Sulaima, M.N.M. Nasir and H.I. Jaafar
Faculty of Electrical Engineering, Universiti Teknikal Malaysia Melaka, Hang Tuah Jaya,
76100 Durian Tunggal, Melaka, Malaysia
Research Journal of Applied Sciences, Engineering and Technology 2014 2:235-242
Received: April 08, 2014 | Accepted: April 28, 2014 | Published: July 10, 2014
Abstract
Electromyography (EMG) is a bio signal that is formed by physiological variations in the state of muscle fibre membranes. Pattern recognition is one of the fields in the bio-signal processing which classified the signal into certain desired categories with subject to their area of application. This study described the classification of the EMG signal based on human body percentile using Self Organizing Mapping (SOM) technique. Different human percentile definitively varies the arm circumference size. Variation of arm circumference is due to fatty tissue that lay between active muscle and skin. Generally the fatty tissue would decrease the overall amplitude of the EMG signal. Data collection is conducted randomly with fifteen subjects that have numerous percentiles using non-invasive technique at Biceps Brachii muscle. The signals are then going through filtering process to prepare them for the next stage. Then, five well known time domain feature extraction methods are applied to the signal before the classification process. Self Organizing Map (SOM) technique is used as a classifier to discriminate between the human percentiles. Result shows that SOM is capable in clustering the EMG signal to the desired human percentile categories by optimizing the neurons of the technique.
Keywords:
Biceps brachii, classification, electromyography, human percentile, pattern recognition, self organizing map,
References
-
Ahsan, M.R., 2011. Electromygraphy (EMG) signal based hand gesture recognition using Artificial Neural Network (ANN). Proceeding of the International Conference on Mechatronics (ICOM), Vol. 4.
CrossRef -
Angkoon, P., L. Chusak and P. Pornchai, 2009. A novel feature extraction for robust EMG pattern recognition. J. Comput., 1(1): 71-80.
-
Bartuzi, P., T. Tomasz and R.L. Danuta, 2010. The effect of the fatty tissue on EMG signal in young women. Acta Bioeng. Biomech., 12(2): 87-93.
PMid:20882946 -
Bohari, Z.H., S. Ab Ghani, M.F. Baharom, M.N.M. Nasir, M.H. Jali and Y.H. Md Thayoo, 2014. Feature analysis of numerical calculated data from Sweep Frequency Analysis (SFRA) traces using self organizing maps. J. Teknol., 67(3).
CrossRef -
Chan, F.H.Y., Y.S. Yang, F.K. Lam, Y.T. Chang and P.A. Parker, 2000. Fuzzy EMG classification for prothesis control. IEEE T. Rehabil. Eng., 8(3): 305-311.
CrossRef PMid:11001510 -
Chiharu, I., S. Satoru, Sasaki and H. Hiroshi, 2012. Distinction of finger operation for myoelectric prosthetic hand on the basis of surface EMG. Proceeding of the 16th CSI International Symposium on Artificial Intelligence and Signal Processing (AISP), pp: 428-434.
-
Frisancho, A.R., 1974. Triceps skin fold and upper arm muscle size norms for assessment of nutritional status. Am. J. Clin. Nutr., 27(10): 1052-1058.
PMid:4419774 -
Graupe, D., J. Magnussen and A.A.M. Beex, 1978. A microprocessor system for multifunctional control of upper limb prostheses via myoelectric signal identification. IEEE T. Automat. Contr., 23(4): 538-544.
CrossRef -
Hamedi, M., S.H. Salleh, A.M. Noor, T.T. Swee and I.K. Afizam, 2012. Comparison of different time-domain feature extraction methods on facial gestures' EMGs. Proceedings of the Progress in Electromagnetics Research Symposium, pp: 1897-1901.
-
Hudgins, B., P.A. Parker and R.N. Scott, 1993. A new strategy for multifunction myoelectric control. IEEE T. Biomed. Eng., 40(1): 82-94.
CrossRef PMid:8468080 -
Jali, M.H., M.F. Sulaima, T.A. Izzuddin, W.M. Bukhari and M.F. Baharom, 2014. Comparative study of EMG based joint torque estimation ANN models for arm rehabilitation device. Int. J. Appl. Eng. Res., 9(10): 1289-1301.
-
Joyce, C., Z. Jane Wang and J.M. Martin, 2008. A Hidden Markov, multivariate autoregressive (HMM-mAR) network framework for analysis of surface EMG (sEMG) data. IEEE T. Signal Proces., 56(8): 4069-4081.
-
Kelly, M.F., P.A. Parker and R.N. Scott, 1990. The application of neural networks to myoelectric signal analysis: A preliminary study. IEEE T. Biomed. Eng., 37(3): 211-230.
CrossRef PMid:2328997 -
Kentarou, N., A. Keiiti, Y. Masahumi and M. Kazushige, 2006. Recognition of hand motions based on multi-channel SEMG employing the Monte Carlo method for channel selection. T. Jpn. Soc. Med. Biol. Eng., 44(1): 138-147.
-
Killer, J.M., M.R. Gray and J.A. Givens, 1986. A fuzzy k-nearest neighbor algorithm. IEEE T. Syst. Man Cyb., 15(4): 580-585.
CrossRef -
Lee, S. and G.N. Saridis, 1984. The control of a prosthetic arm by EMG pattern recognition. IEEE T. Automat. Contr., 29(4): 290-302.
CrossRef -
Peter, K., 2006. The ABC of EMG: A Practical Introduction to Kinesiological Electromyography. Version 1.4, Noraxon Inc., U.S.A.
-
Scott, O. and T. Erin, 2006. Ergonomics and Design: A Reference Guideline. Diane Publishing, New York.
-
Simon, H., 1999. Neural Networks: A Comprehensive Foundation, Prentice Hall, New Jersey.
-
Tsuji, T., D. Mori and K. Ito, 1992. Motion discrimination method from EMG signals using statistically structured neural networks. T. Inst. Electr. Eng. Jpn., 112-C(8): 465-473 (in Japanese).
CrossRef -
Wang, L. and T.S. Buchanan, 2002. Prediction of joint moments using a neural network model of muscle activations from EMG signals. IEEE T. Neur. Sys. Reh., 10(1).
Competing interests
The authors have no competing interests.
Open Access Policy
This article is distributed under the terms of the Creative Commons Attribution 4.0 International License (http://creativecommons.org/licenses/by/4.0/), which permits unrestricted use, distribution, and reproduction in any medium, provided you give appropriate credit to the original author(s) and the source, provide a link to the Creative Commons license, and indicate if changes were made.
Copyright
The authors have no competing interests.
|
|
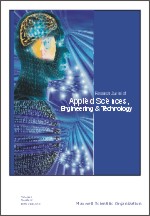 |
ISSN (Online): 2040-7467
ISSN (Print): 2040-7459 |
 |
Information |
|
|
|
Sales & Services |
|
|
|